Pioneering Research
We are dedicated to setting the industry standard in AI technology research, and playing a pivotal role in harnessing voice and language biomarkers for cognitive and mental health assessment and overall well-being.
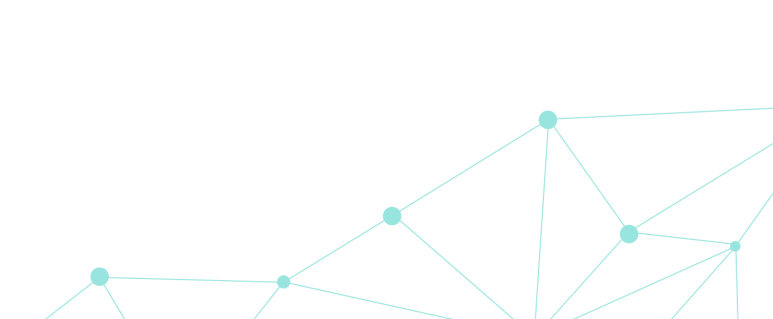
MULTILINGUAL SYSTEM FOR EARLYDETECTION OF NEURODEGENERATIVEAND PSYCHIATRIC DISORDERS
Lang Aware , Inc.
Abstract
The present disclosure provides a system for predicting a disease state based on speech occurrences . A feature extraction module extracts a plurality of lingual features from a speech record of the speech occurrence. The lingual features are chosen based on a correlation between the lingual features and the disease state in at least a first language and a second language . The lingual features are consistent for transcripts in at least the first language and the second language . A prediction module including a trained classification model generates a prediction of the disease state for speech occurrences in at least the first language and the second language using the lingual features extracted from the speech records .
Automatic Detection of Linguistic indicators as a means of early detection of Alzheimer’s disease and of related dementias: A Computational Linguistics analysis
Vassiliki Rentoumi, George Paliouras, Dimitra Arfani, Katerina Fragkopoulou, Spyridoula Varlokosta, Eva Danasi, Spyros Papadatos
Abstract
In the present study, we analyzed written samples obtained from Greek native speakers diagnosed with Alzheimer’s in mild and moderate stages and from age matched cognitively normal controls (NC). We adopted a computational approach for the comparison of morpho-syntactic complexity and lexical variety in the samples. We used text classification approaches to assign the samples to one of the two groups. The classifiers were tested using various features: morpho-syntactic and lexical characteristics. The proposed method excels in discerning AD patients in mild and moderate stages from NC leading to the in-depth understanding of language deficits.
Features and Machine Learning Classification of Connected Speech Samples from Patients with Autopsy Proven Alzheimer’s Disease with and without Additional Vascular Pathology
Vassiliki Rentoumi, Ladan Raoufiana, Samrah Ahmedb, Celeste A. de Jagerc and Peter Garrarda
Abstract
Mixed vascular and Alzheimer-type dementia and pure Alzheimer’s disease are both associated with changes in spoken language. These changes have, however, seldom been subjected to systematic comparison. In the present study, we analyzed language samples obtained during the course of a longitudinal clinical study from patients in whom one or other pathology was verified at post mortem. The aims of the study were twofold: first, to confirm the presence of differences in language produced by members of the two groups using quantitative methods of evaluation; and secondly to ascertain the most informative sources of variation between the groups. We adopted a computational approach to evaluate digitized transcripts of connected speech along a range of language-related dimensions. We then used machine learning text classification to assign the samples to one of the two pathological groups on the basis of these features. The classifiers’ accuracies were tested using simple lexical features, syntactic features, and more complex statistical and information theory characteristics. Maximum accuracy was achieved when word occurrences and frequencies alone were used. Features based on syntactic and lexical complexity yielded lower discrimination scores, but all combinations of features showed significantly better performance than a baseline condition in which every transcript was assigned randomly to one of the two classes. The classification results illustrate the word content specific differences in the spoken language of the two groups. In addition, those with mixed pathology were found to exhibit a marked reduction in lexical variation and complexity compared to their pure AD counterparts.
Machine learning approaches to diagnosis and laterality effects in semantic dementia discourse
Peter Garrard, Vassiliki Rentoumi, Benno Gesierich, Bruce Miller and Maria Luisa Gorno-Tempini
Abstract
Advances in automatic text classification have been necessitated by the rapid increase in the availability of digital documents. Machine learning (ML) algorithms can ‘learn’ from data: for instance a ML system can be trained on a set of features derived from written texts belonging to known categories, and learn to distinguish between them. Such a trained system can then be used to classify unseen texts. In this paper, we explore the potential of the technique to classify transcribed speech samples along clinical dimensions, using vocabulary data alone. We report the accuracy with which two related ML algorithms [naive Bayes Gaussian (NBG) and naive Bayes multinomial (NBM)] categorized picture descriptions produced by: 32 semantic dementia (SD) patients versus 10 healthy, age- matched controls; and SD patients with left- (n 1⁄4 21) versus right-predominant (n 1⁄4 11) patterns of temporal lobe atrophy. We used information gain (IG) to identify the vocabulary features that were most informative to each of these two distinctions. In the SD versus control classification task, both algorithms achieved accuracies of greater than 90%. In the right- versus left-temporal lobe predominant classification, NBM achieved a high level of accuracy (88%), but this was achieved by both NBM and NBG when the features used in the training set were restricted to those with high values of IG. The most informative features for the patient versus control task were low frequency content words, generic terms and components of meta narrative statements. For the right versus left task the number of informative lexical features was too small to support any specific inferences. An enriched feature set, including values derived from Quantitative Production Analysis (QPA) may shed further light on this little understood distinction.
Linguistic biomarkers of Hubris syndrome
Peter Garrard, Vassiliki Rentoumi, Christian Lambert and David Owen
Abstract
Owen and Davidson coined the term ‘Hubris Syndrome’ (HS) for a characteristic pattern of exuberant self-confidence, recklessness, and contempt for others, shown by some individuals holding substantial power. Meaning, emotion and attitude are communicated intentionally through language, but psychological and cognitive changes can be reflected in more subtle ways, of which a speaker remains unaware. Of the fourteen symptoms of HS, four imply lexical choices: use of the third person/‘royal we’; excessive confidence; exaggerated self-belief; and supposed accountability to God or History. One other feature (recklessness) could influence language complexity if impulsivity leads to unpredictability. These hypotheses were tested by examining transcribed spoken discourse samples produced by two British Prime Ministers (Margaret Thatcher and Tony Blair) who were said to meet criteria for HS, and one (John Major) who did not. We used Shannon entropy to reflect informational complexity, and temporal correlations (words or phrases whose relative frequency correlated negatively with time in office) and keyness values to identify lexical choices corresponding to periods during which HS was evident. Entropy fluctuated in all three subjects, but consistent (upward) trends in HS-positive subjects corresponded to periods of hubristic behaviour. The first person pronouns ‘I’ and ‘me’ and the word ‘sure’ were among the strongest positive temporal correlates in Blair’s speeches. Words and phrases that correlated in the speeches of Thatcher and Blair but not in those of Major included the phrase ‘we shall’ and ‘duties’ (both negative). The keyness ratio of ‘we’ to ‘I’ was clearly higher throughout the terms of office of Thatcher and Blair that at any point in the premiership of Major, and this difference was particularly marked in the case of Blair. The findings are discussed in the context of historical evidence and ideas for enhancing the signal to noise ratio put forward.
The acute mania of King George III: Acomputational linguistic analysis
Vassiliki Rentoumi, Timothy Peters, Jonathan Conlin, Peter Garrard
Abstract
We used a computational linguistic approach, exploiting machine learning techniques, to examine the letters written by King George III during mentally healthy and apparently mentally ill periods of his life. The aims of the study were: first, to establish the existence of alterations in the King’s written language at the onset of his first manic episode; and secondly to identify salient sources of variation contributing to the changes. Effects on language were sought in two control conditions (politically stressful vs. politically tranquil periods and seasonal variation). We found clear differences in the letter corpus, across a range of different features, in association with the onset of mental derangement, which were driven by a combination of linguistic and information theory features that appeared to be specific to the contrast between acute mania and mental stability. The paucity of existing data relevant to changes in written language in the presence of acute mania suggests that lexical, syntactic and stylometric descriptions of written discourse produced by a cohort of patients with a diagnosis of acute mania will be necessary to support the diagnosis independently and to look for other periods of mental illness of the course of the King’s life, and in other historically significant figures with similarly large archives of handwritten documents.
Join us in revolutionizing healthcare.
Let’s partner!